Are Hong Kong's woes good for Singaporian data science?
Plus: AI-startup hype smell a lot like Theranos
AltDeep is a newsletter focused on microtrend-spotting in data and decision science, machine learning, and AI. It is authored by Robert Osazuwa Ness, a Ph.D. machine learning engineer at an AI startup and adjunct professor at Northeastern University.
From last week
Hong Kong’s troubles may boost Singapore, and by extension its data science community
WSJ singles out Engineer.ai for Theranos-style hyping of AI tech
A prediction market for betting on reproducible research
Signals from China
Hong Kong’s troubles may boost Singapore, and by extension its data science community
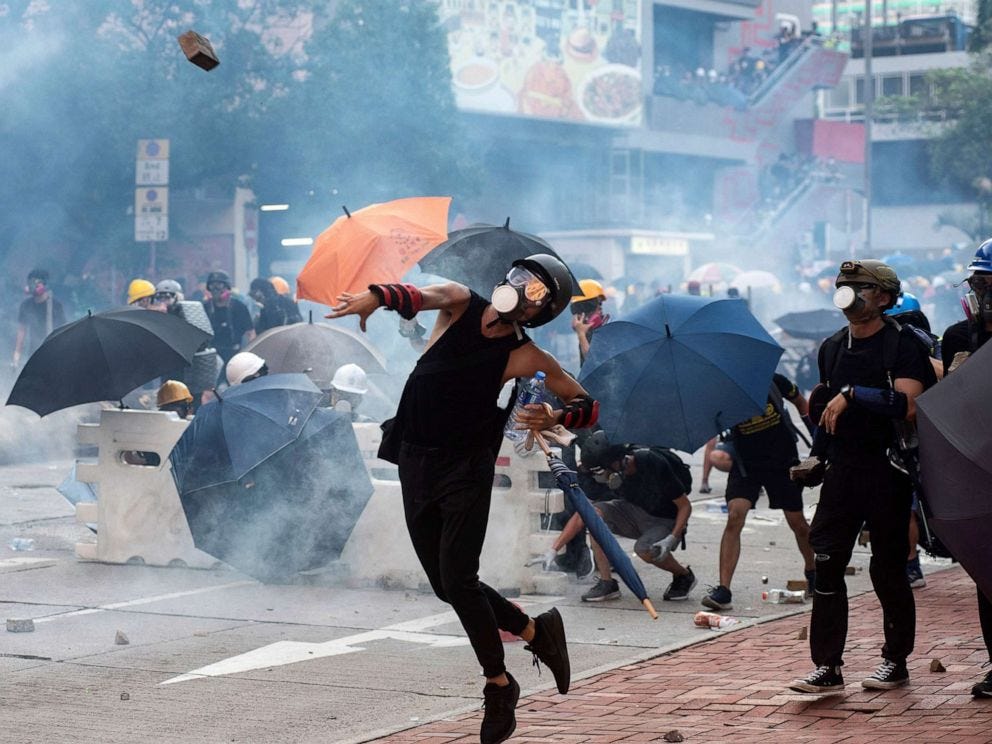
Hong Kong has entered an 11th week of massive protests. The People's Liberation Army will likely get involved. International firms are already looking for other places to plant APAC investment.
Singapore stands up as a top choice. Earlier this year, Savills Tech Cities index ranked it as first in Asia, and sixth globally, after NYC, SF, London, Amsterdam, and Boston. Most of the U.S. tech giants have offices in Singapore. In the past roles in these Singapore offices have skewed towards communications and marketing roles, but data scientist and machine learning roles do pop up (e.g., Facebook, Google). Local tech companies, such as Grab, more aggressively hire for these roles. Of course, financial institutions are other major employers of these roles.
If APAC investment shifts from Hong Kong to Singapore, it could add inertia to the growth of data science in the city-state.
But, but, but: China is still dead serious about dominating the world in AI. To see just how serious they are, read this English translation of a Chinese Ministry of Industry and Information Technology-sponsored report on the global AI industry.
Ear to the Ground
DeepMind’s losses and billions in debt invites deep reinforcement learning skepticism
Gary Marcus chimed in on recent reporting of DeepMind’s 100’s of millions of losses and billions in debt. If you are familiar with Gary Marcus’s opinions, nothing here is surprising. However, if you are unfamiliar with his skepticism about deep learning, this is worth the time, as it talks specifically about DeepMind and the economics of research into deep reinforcement learning.
Background. Reinforcement learning means training a decision-making agent by rewarding it for desired performance (as opposed to giving it many examples of correct decisions as in supervised learning). It has seen growing interest from the ML research community, but commercial applications remain elusive.
DeepMind is an Alphabet company that focuses on applying deep learning to the reinforcement learning problem. With this technique, they beat top Go champions and professional video game players.
Gary Marcus is an ML researcher and was a co-founder of an AI startup that was acquired by Uber. Marcus is famous for a clever PR hustle that involves starting arguments with famous deep learning people on Twitter, often with minimal participation from the other side, and then citing the “debate” in articles he writes. That said, I always find myself agreeing with the guy.
WSJ singles out Engineer.ai for Theranos-style hyping of AI tech
Engineer.ai says its “human-assisted AI” allows anyone to create a mobile app by clicking through a menu on its website. Users can then choose existing apps similar to their idea, such as Uber’s or Facebook’s. Then Engineer.ai creates the app largely automatically, it says, making the process cheaper and quicker than conventional app development.
Documents reviewed by The Wall Street Journal and several people familiar with the company’s operations, including current and former staff, suggest Engineer.ai doesn’t use AI to assemble code for apps as it claims. They indicated that the company relies on human engineers in India and elsewhere to do most of that work, and that its AI claims are inflated even in light of the fake-it-till-you-make-it mentality common among tech startups.
I don’t make my Theranos comparison likely. Theranos demonstrated that while the tech industry’s fake-it-till-you-make-it culture might work for software, it doesn’t work when building cutting-edge medical nanotech. I suspect the same is true for SpaceX’s rocket ships, or with quantum computing — it is harder to bullshit people on hardware.
AI is somewhere in between. It’s generally more about algorithms than physics, but it is still a hardware problem at large scale. With software, you often can rely on some agile development process or lean-startup methodology to deliver features you already promised investors were near completion. However, automating workflow with AI in software requires a bespoke problem-solving effort that looks a lot like actual scientific research. Trying to shoehorn research into lean startup framework is, in my experience, folly.
Data Sciencing for Fun and Profit
A clever application of a prediction market to addressing the reproducibility crisis in social and behavioral science. You can bet and win cash on whether researchers can reproduce the published findings of 3,000 experiments.