Last week…
…I said this to people;
Dear AI startup CEO: Have an AI philosophy
AI startups should have an AI philosophy that biases engineers toward a specific class of solutions. In my current gig, it is the idea of AI as “Bayesian program synthesis,” which I like. At my last gig, it was “radical empiricism,” which I hated (because data can't speak for itself, damn it. Plus, stop putting “radical” in front of things everyone accepts are good and calling it profound.) But even that was better than nothing.
The philosophy should be articulated by AI experts working with go-to-market experts, match the nature of the data, and not feel like copy-pasta from a Forrester report or Wired article.
And for the young bucks…

Subscribe to the paid edition
The paid edition contains more in-depth topical posts, more actionable trend signals, and some data analysis
This week I share some insight on a new and interesting metric investors are tossing about about when evaluating AI startups with natural language processing as core tech.
Ear to the Ground
Curated posts aimed at spotting microtrends in data science, machine learning, and AI.
Essential reading for future VPs of Eng. in AI companies
I typically shy away from engineering architecture posts but this can’t be ignored.
Few companies rival Uber as exemplars of modern machine-learning-powered logistics. It is a setting where a small army of data scientists and engineers need to train and deploy models for various tasks.
Now the company has published an evaluation of the first three years of operations of its machine learning platform Michelangelo and it’s worth a read.
That said, there is nothing surprising here for those with some data engineering experience. What is important here is the level of detail; this is essential reading for someone involve in building something similar.
Machine learning as software engineering. One thing that jumped out at me; Uber puts a strong emphasis on the idea that engineering machine learning is a software engineering process. In my experience, this is fine for when inputs and outputs are fixed, and no novel machine learning solutions need inventing.
But sometimes you have to do something novel and need to do actual research. The only difference from academic research is that the goal is a bespoke research solution specific to needs of an individual business, as opposed to a general solution with broad impact that might lead to academic publication. Bespoke research is still research, and there is a reason research labs don’t typically use software development frameworks to organize their work.
Evolving Michelangelo Model Representation for Flexibility at Scale — Uber Engineering Blog
OpenAI uses AI with robotic hand to solve Rubik’s cube
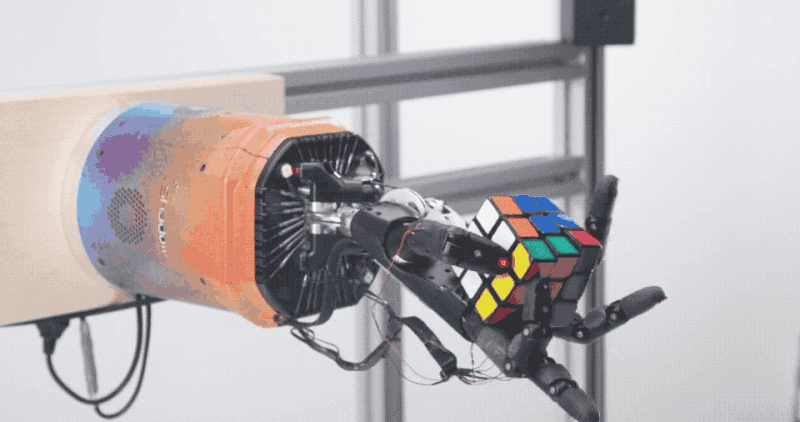
This made the rounds because of a cool viral video. The accomplishment here is not using AI to solve a Rubik’s cube (this is a solved problem). Rather, the achievement is using AI to guide a mechanical hand in physically manipulating a Rubik’s cube to the solution. This is AI-powered dexterity. The robot hand was general-purpose rather than custom-built for solving Rubik’s cubes. Further, the algorithm used reinforcement learning (a Pavlovian way of teaching algorithms that learn) to train the algorithm in a simulated environment. The training results were then transferred to the physical machine. This manner of training robots has long been a (rarely delivered upon) promise of reinforcement learning.
Some important context:
This approach only works for Rubik’s cubes. It would not extend to, say, opening puzzle boxes.
Physical failures, such as dropping the cube, were common.
Resources:
Solving Rubik’s Cube with a Robot Hand — OpenAI Blog
Modeling AI based on a 4-year old’s brain
Interesting podcast episode (with transcript) that interviews developmental psychologist Alison Gopnik:
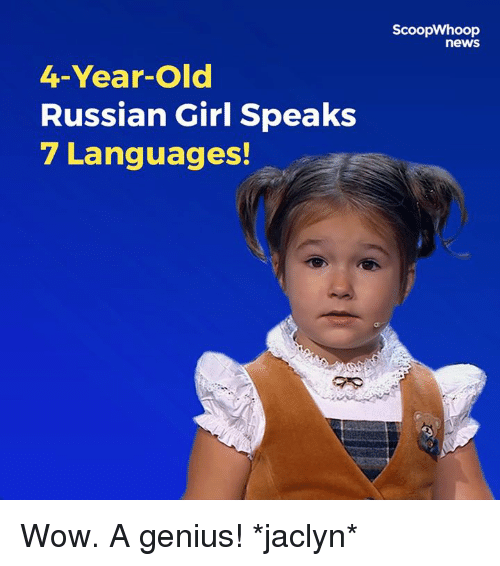
…you see this pattern of development where you start out with this very plastic system with lots of local connection, and then you have a tipping point where that turns into a system that has fewer connections but much stronger, more long-distance connections…
It’s interesting that that isn’t an architecture that’s typically been used in AI…
You can have a lot of random search, or you can solve a problem that’s very highly constrained, but the combination of being able to solve problems that are highly constrained and search for solutions that are further away has been the most challenging problem for AI to solve. That’s a problem that children characteristically solve more effectively than adults.
Signals from China
News on China AI, with the China fear filtered out.
Relying on the valley to compete with China
The New York Times published a piece about America’s reliance on the Silicon Valley tech giants to compete with China’s big state-funded AI initiatives.
I am unconvinced. China has a long history of poorly choosing champions in industrial policy. Huawei, Alibaba, and Lenovo are all companies that succeeded despite the Party’s intercession, not because of it.

That said, the article has some good advice on support US AI development through immigration policy.
America’s Risky Approach to Artificial Intelligence — The New York Times
Publish or impoverish
Some trend reporting on China’s wide-spread policy of giving monetary rewards for publication.
A landscape of the cash-per-publication reward policy in China emerged as all 168 cash reward policies were analyzed. Chinese universities offer cash rewards that range from 30 to 165,000 USD for a single paper published in journals indexed by WoS, and the average reward amount has been increasing for the past 10 years.
Quan, Wei, Bikun Chen, and Fei Shu. "Publish or impoverish: An investigation of the monetary reward system of science in China (1999-2016)." Aslib Journal of Information Management 69.5 (2017): 486-502.
AI Long Tail
Pre-hype AI microtrends.
After Grammarly valuation, emerging crop of AI writing assistants
These new ones seem focused on SEO suggestions, as opposed to typos and grammar. Ink is the second of such apps I’ve written about here.
Related:
Acciyo uses natural language processing to organize news stories into a timeline.
Acciyo populates an interactive, zoomable timeline of previous articles published on the subject you're currently reading. It's finally possible to binge-read a topic in the news.
The Economist chimes in on ghost work startups
The Economist has a full article on the growth industry of AI startups that handle the unglamorous task of data-labeling.
Hive has turned data-labelling into something “like playing Candy Crush”, explains its boss, Kevin Guo, referring to a hit tile-matching game. Its mobile app makes it easy for users to identify objects, earning money instead of points.
If you enjoy this article, I recommend reading the book Ghost Work. I found this book well-researched, and I agreed with half the points while disagreeing with the other half. Good books do that.
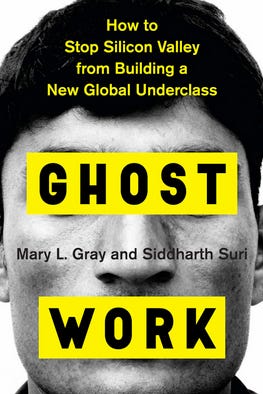
Thanks for your support. Like what you see? Why not send a gift subscription?