Modeling of fat-tailed COVID-19 is folly
On social and data scientist efforts to model. Plus, the end of Hong Kong
Ear to the Ground
Landlords are Zucked — Must-read article on Facebook’s remote work announcement and future of office space from Dror Proleg, whose newsletter on commercial real estate is freaking fantastic.
A Massive Opportunity Exists To Build “Picks And Shovels” For Machine Learning — Articulates the argument that there are startup opportunities for ML-DevOps microservices. It’s not the first time I’ve heard the argument, but it doesn’t really resonate with me quite yet.
Machine Learning Engineers will not Exist in 10 Years — Interesting analysis of the machine learning engineer role, though obviously nothing in this space will be the same in 10 years.
Curing Coronavirus May Not a Job for Social Scientists…
At the start of the month, Anthony Fowler wrote an op-ed criticizing the response of social scientists to Covid-19. Andrew Gelman, a prominent statistician and outspoken critic of crappy research, backs him up.
The central argument is that all of the pathologies that plague the social science research (see Gelman’s work here and here) are exacerbated by the public’s appetite for COVID-19-related information, such that the result is a possible public harm.
He suggests numerous studies on how people of different political stripes respond to the pandemic display bad methodology. For example, several groups have studied the causal effect of conservative vs. liberal bias on social distancing compliance. However, both one’s political bias and the practicalities of social distancing depend on where you live (urban vs. rural), and these studies often fail to control for this obvious confounder. Moreover, the studies are often just salacious:
Another recent study [parodied here] investigated the extent to which watching “Hannity” versus “Tucker Carlson Tonight” may have increased the spread of Covid-19. This is the kind of study that might make one skeptical in normal times. An extra concern now is that the paper was likely written in just a few days. Although the authors write that they used variation in sunset times to estimate the effect of watching “Hannity,” a closer reading suggests that they’re mostly using variation in how much people in different media markets watch television and how much Fox News they watch. Maybe conservative commentators like Sean Hannity have exacerbated the spread of Covid-19, but it’s dangerous for social scientists to publicize these kinds of results before they have been carefully vetted.
Curing Coronavirus Isn’t a Job for Social Scientists — Bloomberg
Here’s what academic social, behavioral, and economic scientists should be working on right now. — Andrew Gelman’s blog
2 perspectives on the relevance of social science to our current predicament: (1) social scientists should back off, or (2) social science has a lot to offer — Andrew Gelman’s blog
…but is it a job for data scientists?
I generally agree with Fowler. Interestingly, it reminds me of some recent Twitter sentiment suggesting data modelers should not be involved in modeling Covid-19.
Fellow probabilistic programming developer Thomas Wiecki posted this question back in April.
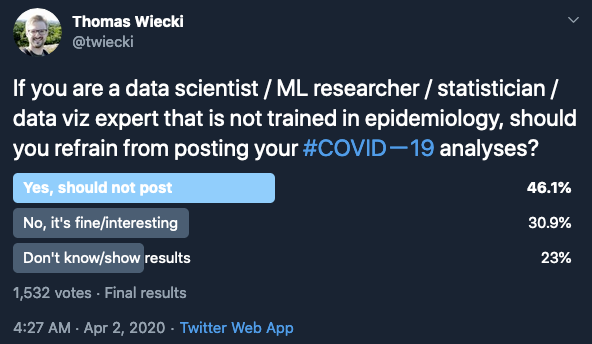
This is bonkers to me. Firstly, the whole point of statistics is providing general methods for data modeling across domains. Likewise, the whole point of a statistician is having a non-domain expert who knows the ins-and-outs of modeling to help domain experts model. Wiecki himself took a model built by epidemiologists and integrated it into a Bayesian framework that accounted for location (see my interview with him on the topic). The skillset required to both understand, implement, and train such models are rare and should be so because domain experts should be too busy mastering their domain to learn it.
“The lowest point in the 74-year history of the Council of Economic Advisers”
But Thomas Wiecki is a talented modeler. Some people suck at modeling. For example, economist Kevin Hassett of the Council of Economic Advisers has now become the poster boy of the argument that social scientists should sit this one out. He did a tragic curve-fitting exercise called a “cubic fit”.

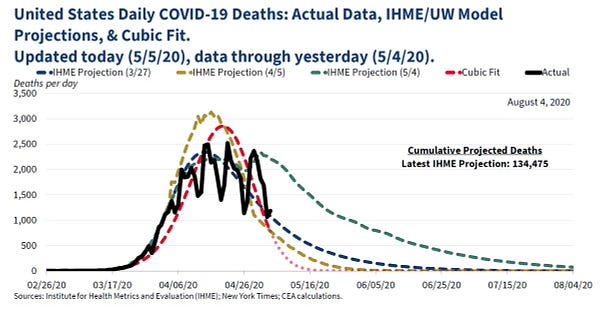
This plot overlays the red “cubic fit” line with several outdated forecasts to make it look sane. For background, in mathematics, a cubic function is characterized by multiple inflection points.
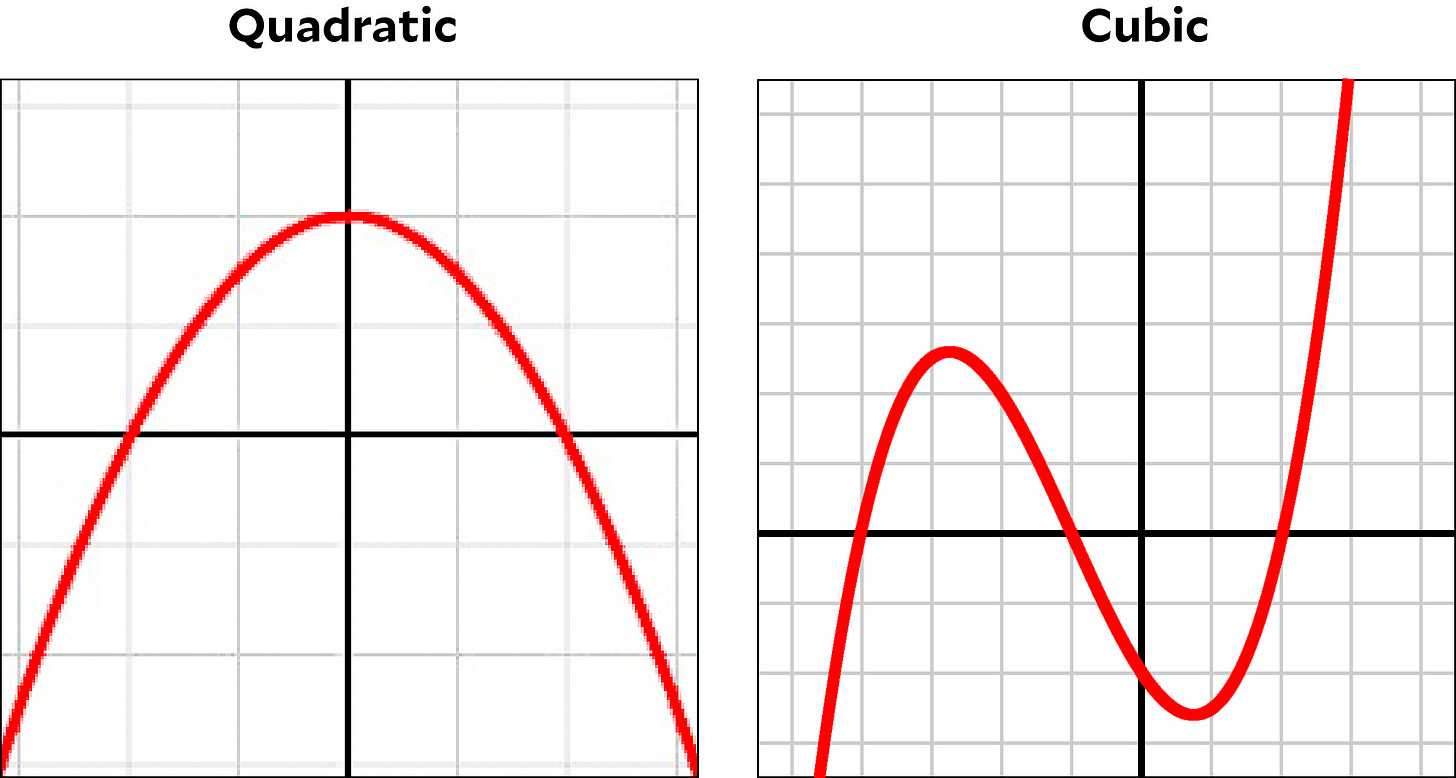
Long story short, one does not look at this data and think “multiple inflection points, let’s fit a cubic function!”
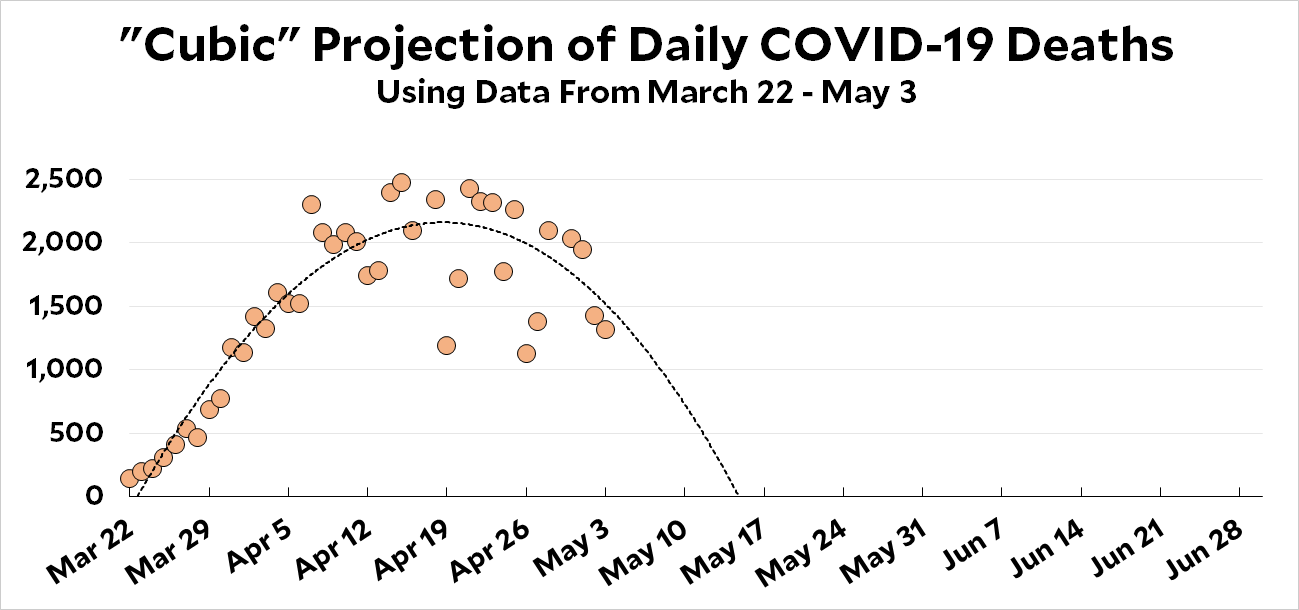
To understand how insane this modeling choice was, scan the replies to the above tweet. The only justification is that Hassett wanted to provide a plot that made the big man happy.
Hassett’s blunder makes it clear we have no way of knowing which model to trust. Our culture relies on the authority of well-credentialed individuals in prestigious jobs at elite institutions to help us understand which empirical conclusions to believe. While one might argue the current degree of White House dysfunction is a singular example, this epidemic has made it clear that we lack general mechanisms in finding trustworthy modelers. All this Twitter COVID-19 data science, even from folks we may respect personally, is starting to feel like noise.
Lying With Statistics, COVID-19 Edition — Mother Jones
“This might be the lowest point in the 74 year history of the Council of Economic Advisers.” — Jason Furmon’s tweet thread
Data science can’t handle fat tails
Putting aside the competence of the modelers themselves, the things they may be trying to do with their modeling may be mathematically impossible.
Like this post? Please share it with a friend.
For example, many models focus on estimating the basic reproduction number, AKA R0 or Rt, the average number of people infected by one person at a given point in time.
This concept represents the mean of a fat-tailed distribution, which means that for an R0 1.4, there are still many super-spreaders infecting upwards of 80 people.
The problem is that in fat-tailed distributions, statistical estimation theory breaks down, even with large data. Moreover, depending on how fat the tail, a mean like Rt may not even exist. In a forthcoming paper in Nature Physics by Nassim Taleb, he and is co-author argue that historic evidence that this is the case.
The implication is stark; every future pandemic has a decent amount of probability in the fat tail of wiping out much or all of the human population.
Less dramatically, it suggests that even when we avoid “cubic fits”, use the models epidemiologists tell us to use, fit them on good unbiased data derived from ubiquitous diagnostic testing, and use advanced statistical techniques, it might still be folly.
Consider Rt.live, which visualizes local Rt estimates.
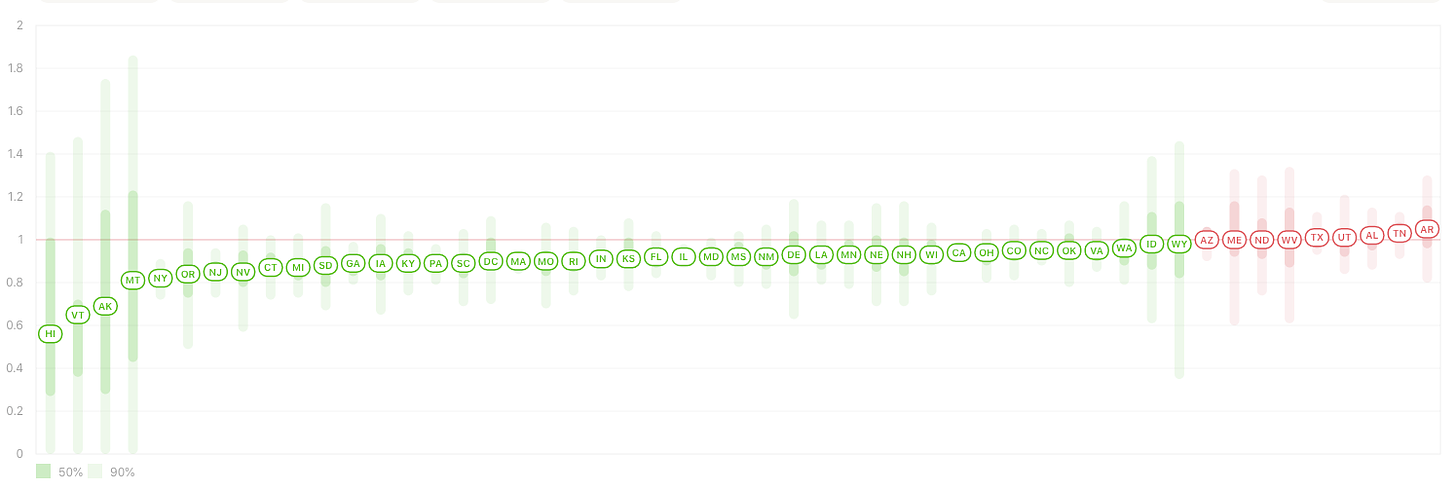
This plot is extremely compelling because when Rt falls below 1, it suggests the number of infections is on a downward spiral. I’ve seen stats people on Twitter yas-queening over this plot and how useful it will be once it incorporates mobility data and better testing data. But if the tail is fat enough, i.e., there are enough super-spreaders infecting upwards of 80 people, then the plot is useless.
One suggestion, report quantiles instead of the Rt mean.
Cirillo, Pasquale, and Nassim Nicholas Taleb. "Tail risk of contagious diseases." arXiv preprint arXiv:2004.08658 (2020).
Signals from China
Stick a fork in her; Hong Kong is done as an Asian financial hub. This will shift a tremendous amount of investment and talent to Singapore, already the APAC center for tech, data science, and ML.
In other news, critics of the stay-at-home orders had argued that it is a trade-off between lives and livelihood. This is a false dichotomy. Firstly, as we’ve seen pandemic deaths come from fat-tailed distributions, so the second a new pandemic surfaces, the best choice is to shut things down immediately until we know it’s not “the big one.”
Unfortunately, that seems beyond our abilities for collective action. So we are down to pricing economic loss from stay-at-home vs. economic loss from contagious infection and death.
China is still selectively shutting down cities. It makes me wonder if they have some internal economic calculus of death and productivity.
Thanks for reading. Leave a comment if you have thoughts.