My sister has COVID-19 but she's not being counted
The numbers are junk and we keep pretending they are not.
Statisticians have invented many wondering mathematical methods for estimation and inference.
However, no statistical method can fix bias in the data. As they say, “junk in, junk out”.
If there is bias in your data, it is better to do nothing than to do analysis and report the results. This is especially true if decision-makers rely on your expertise and the mathematical sophistication of the method as proof of the soundness of the analysis.
However, “expert” data modelers seem not to have gotten the message. They make forecasts on crap data.

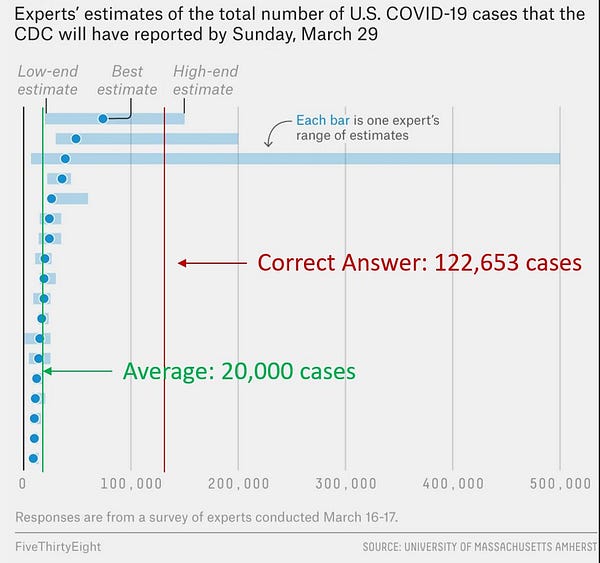
Last week, my sister got diagnosed with COVID-19. She can’t get tested.

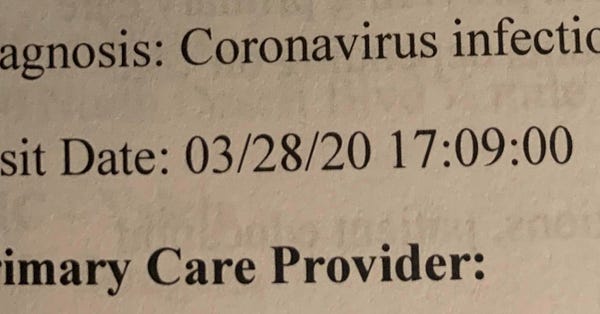
My sister’s scenario is mind-boggling to me. She is fully symptomatic, literally has a COVID-19 diagnosis, as well as chronic asthma, and yet they will not waste a precious test on her. She will not be counted in her state’s statistics.
I find myself wondering, what is the point of testing then? We don’t use it to detect and quarantine the sick. We don’t use it to get a representative percentage of infected individuals in the population.
We just use them to produce junk data, which drives junk journalism and policy-making.
This has been one of my main takeaways so far in this crisis.

And don’t get me started on China…
For example, why do journalists treat Chinese numbers as though they are real despite the evidence that they are not? CNN now treats statements coming from the CCP with less skepticism than statements from the White House. I suppose the former is just less clumsy about its fake news.

Financial analyst friends in China taught me that they never look at reported numbers from the government or Chinese corporations. They always model with some sort of proxy variable.

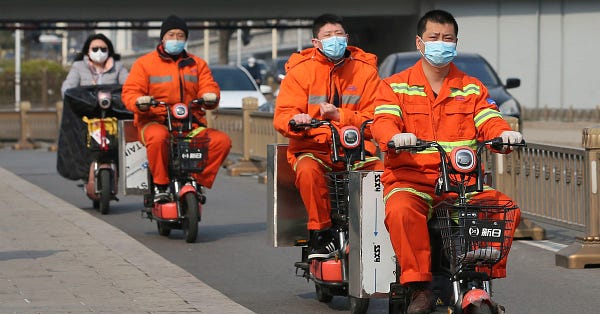
If you want to get a true impression of the numbers in China, just look at the lines of bereaved in front of the crematoriums, waiting to collect family members’ remains. Do the math. How many ovens? How many can corpses can they process a day? How many crematoriums in one city?
Spoiler alert; the math works out to way more than the number of deaths China is reporting.
This is not to say we shouldn’t model
Here is an interview on probabilistic modeling I did with Thomas Wiecki, VP of Quantopian this week.

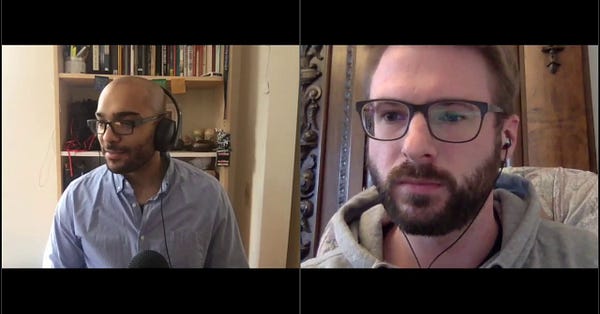
A data scientist can take an epidemic model and train it on all that biased data. On the one hand, that is useful because it takes the subject domain knowledge of experts, encodes it into a computable artifact, and calibrates it on data. This gives us something we can work with.
Most importantly, these models can be used for sensitivity analysis, where you can test how sensitive your model’s forecasts are to bias in the data.