The myth of objective data in the AI fairness debate
A critique of scientific objectivism in the machine learning community.
Tomorrow morning at 11AM EST is my webinar on causal modeling in machine learning. Here is what you’ll get out of it:
An actionable overview of causal inference and modeling using language and examples from machine learning
Access to coding tools that you can use immediately
Hope to see you there!
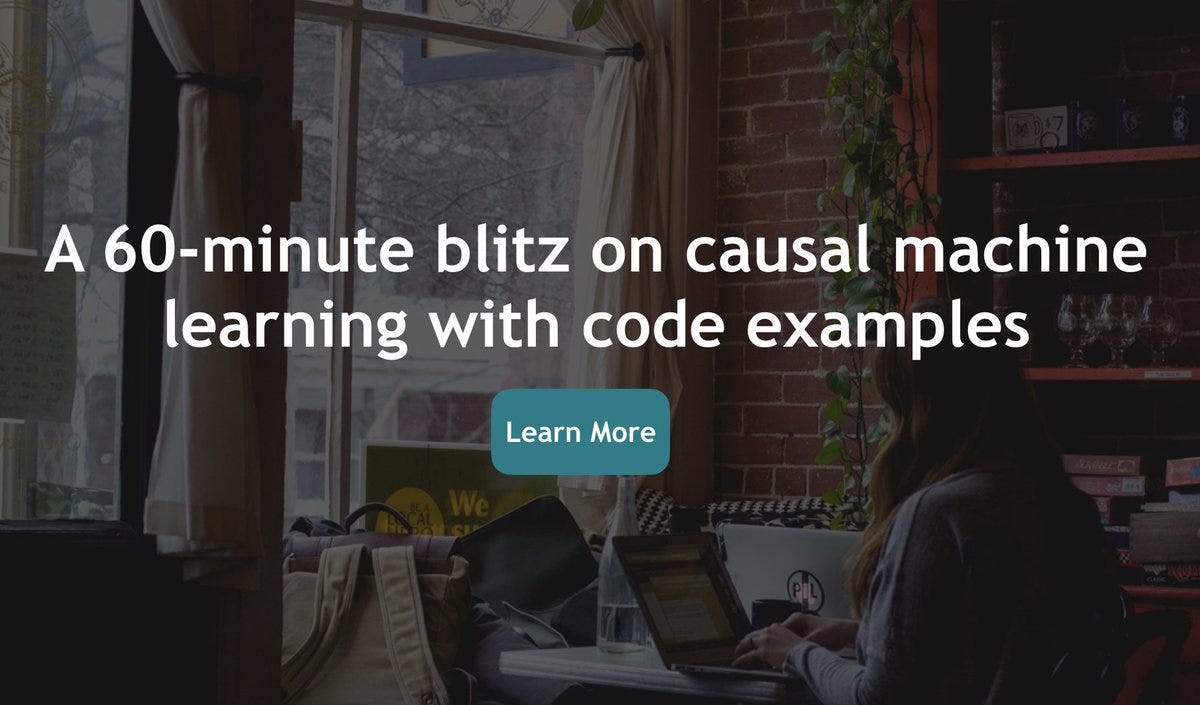
Aside from that, I’m taking a break from webinar prep to quickly rant about scientific objectivism in the AI fairness debate.
There is a common refrain from those who suggest concerns about AI fairness are overblown. It is the idea that data is “objective”.
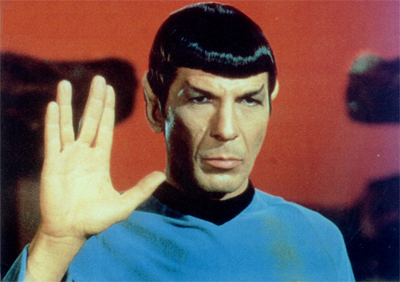
This is a manifestation of scientific objectivism, a value system that is common among academics as well as popular intellectuals like Sam Harris.
From the Stanford Encyclopedia of Philosophy;
Scientific objectivity is a characteristic of scientific claims, methods and results. It expresses the idea that the claims, methods and results of science are not, or should not be influenced by particular perspectives, value commitments, community bias or personal interests, to name a few relevant factors. Objectivity is often considered as an ideal for scientific inquiry, as a good reason for valuing scientific knowledge, and as the basis of the authority of science in society. [emphasis added]
In other words, scientific objectivism is the idea that for a given scientific question, there is (1) an objective truth, and (2) that truth can be inferred from data using reason and empirical analysis. Further, it argues that (3) the scientist's goal should be the discovery of that truth, without consideration of whether it clashes with your religious dogma, your ideology, or standards of political correctness.
I’m a critic of scientific objectivism for the following reasons. (1) Often "objective truth" is subjectively defined by how we pose the question. What is the “true” value of the “rate of unemployment” that puts us at “full employment,” when those things are macroeconomic abstractions we made up and which are defined in terms of other macroeconomic abstractions we made up. If you think this sounds like semantic quibbling, I suggest you read my write-up on Goodman's New Riddle of Induction — the gist is that you can come up with some pretty wacky yet true logical statements just by playing around with the definitions of the abstractions in the premisses of those statements.
(2) Many truths cannot be inferred from data. This is not just typical philosophical issues about induction, but it's also just plain math. The data might simply be insufficient to identify the statistical quantity that answers your question. This is called the parameter identification problem in statistics and econometrics.
(3) Science and politics are inseparable because human biases drive the doing of science. The most straightforward example of this is that the funding of science is always political. No smart scientist fails to consider the politics of a grant-providing agency when they are applying for that grant. More generally, people are going to be biased against things that disconfirm their world view, as well as against new conclusions that conflict with a body of work that underpins their professional reputation.
I have observed that objectivists rely on their supposed objectivism to smoothly transition from conclusions to policies based on those conclusions. “Rigorous analysis of the data shows that this ethnic group is intellectually inferior. Hey guys, I know it’s uncomfortable to hear, but what can I say? Science is science. Oh, by the way, we should block this group from immigrating.”
Scientific objectivism, applied to the data or to empirical inquiry in general, is a fallacy. We in the machine learning community should put it aside.
Thanks for hearing me out. If you think I’m not completely full of it, click subscribe so we can keep the conversation going.