Trump shows how NOT to lie with statistics
4 data science takeaways from Trump's Axios interview
On Monday, President Trump sat down with Axios's Jonathan Swan. I found the most interesting part of the interview was the back-and-forth on COVID-19 statistics. This portion of the interview starts 13 minutes into the video, and is sure to spawn many memes. It also provides us with some insights on how to be better data scientists and data-driven communicators.
How not to lie with statistics
The best-selling statistics book in the second half of the 20th century was "How to Lie with Statistics" by Darrel Huff.
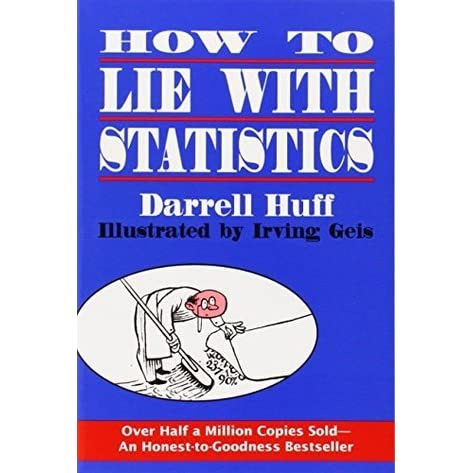
In contrast, this interview felt more like a lesson on "How NOT to Lie with Statistics." Here are some takeaways for anyone seeking to better communicate arguments with data.
1. Nice figures aren't sufficient to make a good argument.
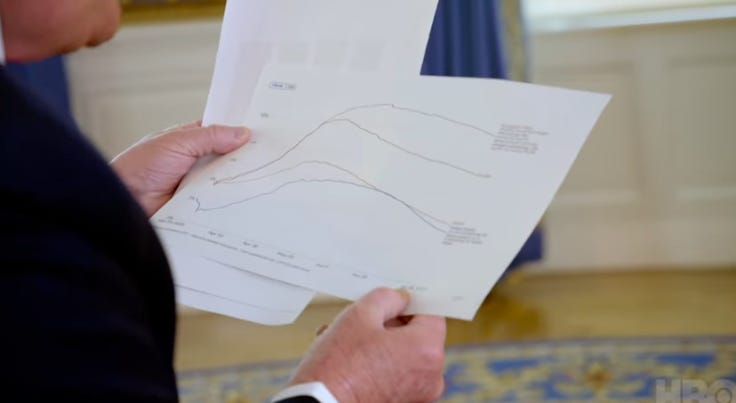
Trump made a common error of presenting figures he ultimately couldn’t explain well. Data can't speak for itself. Visualizations can’t speak for the data. Visualizations only help humans speak for the data. They are a component of an overall presentation.
Moreover, including visualizations usually means you need to rehearse more than you would if you hadn’t included them. Trump seemed to take a "don't take my word for it; just look at the charts" strategy. That is never a good idea.
2. Presentation matters.
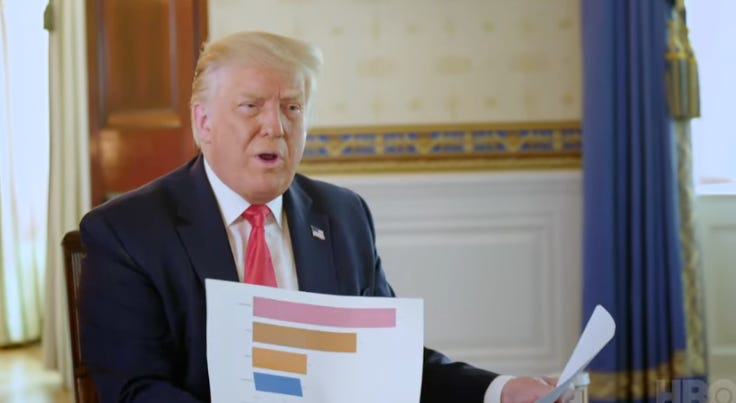
Imagine if Trump's staffers had laminated those plots, or stuck them in plastic sleeves. The parts of the interview where Trump is finding the right plot to support a point he was verbalizing, handing the plots over to Swan, and showing the plots to the camera would have all gone much smoother. Instead, we see cringy moments of an agitated Trump clumsily shuffling his very unlaminated pieces of paper. At the same time, the camera struggles to get a clear shot of the plots, while Swan asks about another set of statistics entirely.
Given Trump's background in television, I'm surprised he made this error.
3. Anticipate statistical counterarguments.
Trump's desire to emphasize statistics that look like a win and downplay statistics that make his administration look bad is understandable. His failure to anticipate Swan's focus on the latter is less so, especially when you know those bad numbers have more emotional impact; "I'm talking about death!" "1000 Americans are dying a day!" counters Swan.
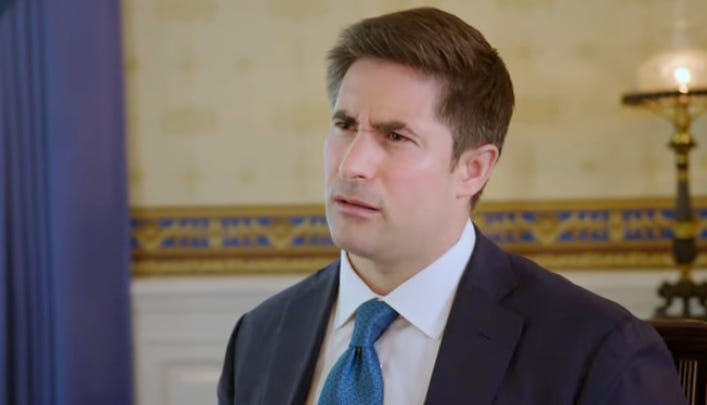
To draw a comparison, imagine being a CEO giving an all-hands to employees and touting recent improvements in sales. Now imagine that this is the first all-hands meeting since a painful period of layoffs. If the CEO did not address the layoffs, and worse yet, was visibly caught off guard when asked about layoffs, you would think poorly of that CEO’s judgment.
4. If you draw causal conclusions, they have to at least make sense.
Let’s put aside the question of whether it is valid to make causal inferences from correlations in data. People will still do it. However, when they do it, the causal inferences they make have to hold water.
Trump focused on the death rate among COVID-19-positive cases in the US. He did so because the number is lower than in other countries, and so he wants to treat it as an indicator of his administration's performance.
However, Trump and Swan compare the US against South Korea and Germany. To convincingly argue that differences in the death rate among COVID-19-positives between countries are evidence of the US administration's good performance, you need to make the following causal assertion: "The US government’s COVID-19 response is better at saving lives of infected people than South Korea’s and Germany’s responses."
That conclusion is unconvincing, given common knowledge that South Korea and Germany are developed countries known for good governance, healthcare, and technological infrastructure. If Trump wants to take credit for this statistic, he would need to convincingly argue why our common knowledge is wrong, and explain how US COVID-19 policy and medical treatment saves more lives than in Korea and Germany. Of course, he couldn’t have done that, so he should not have tried to pump that stat. At least not to an interviewer like Swan.
The President also makes a common and subtle statistical error. He assumes the rate of death among people who tested positive is as an unbiased statistical estimator for the probability of dying if one is infected with COVID-19.
There is the question of test error rates (false positives and false negatives). More importantly, Trump's assumption would be valid if tests were conducted by random sampling. Of course, nobody is testing at random. Nor should they. A government’s goal should be to do selective testing as part of a strategy to contain and manage the pandemic. In the interview, Trump himself boasts about the sophistication of the US testing regime. Sophistication means non-random.
5. Inferences should be consistent.
The President celebrates the lower mortality rate among people who tested positive. He boasts that this is a consequence of more widespread testing. At the same time, Trump has repeatedly called for less testing. That kind of contradiction never helps one make a point.
Numerate people need more numerate newsletters. Help me grow this letter by sharing this email with a friend or colleague!