Two things you might have missed in last weeks AI-bias kerfuffle
Go ahead, click. I promise you won't be outraged.
Ear to the Ground
DON’T CLOSE THIS EMAIL! If you’ve already seen the Obama pic below, you are probably exhausted, and inches away from closing this email and maybe even unsubscribing.
I promise I have something insightful and non-outrage inducing to say about the matter. Just bear with me.
In case you missed it, here’s what happened
It started with this provocative image of President Obama getting "upsampled" into a white man made the rounds on social media. The image comes from the PULSE algorithm.
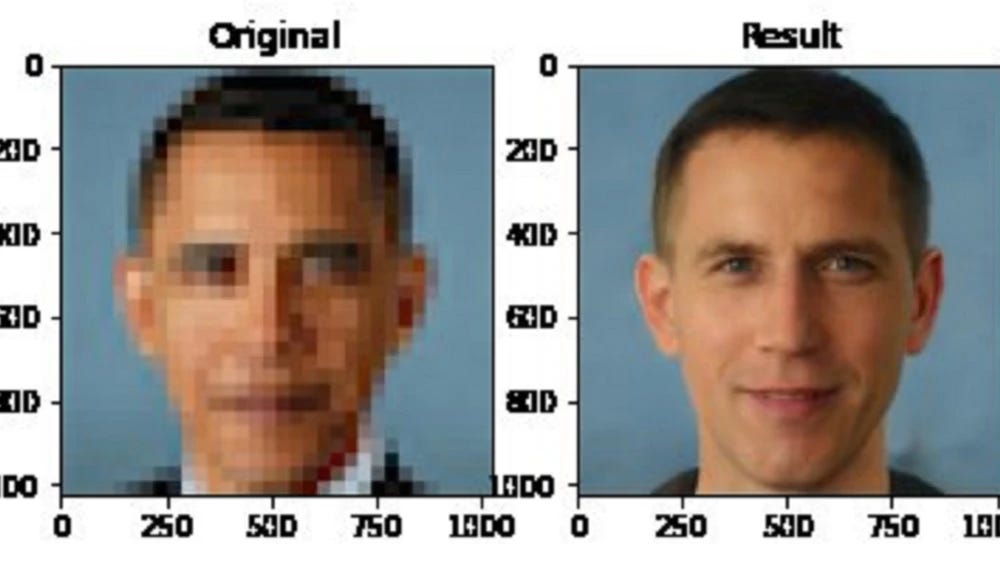
Then yours truly downloaded the algorithm and made a few of his own generated pics, which also made the rounds.

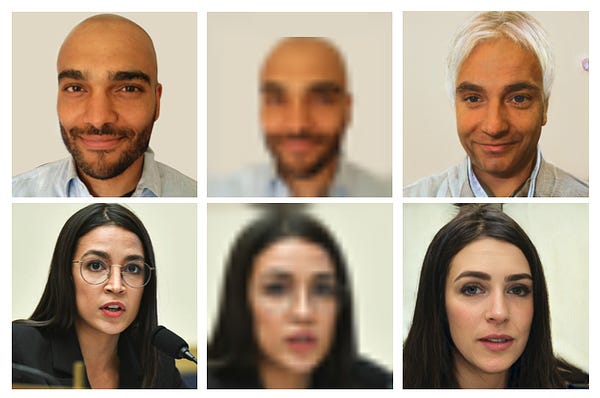
The whole thread and the comments are worth viewing, as I pasted a few other pics of non-white people getting white-ified.
These threads sparked an online debate about algorithmic bias and racism in the machine learning community in general. Yann LeCun, head of Facebook AI and recipient of the Turing award for his work in deep learning, posted the following.


This tweet got some push back by Timnit Gebru. Timnit is a lead on the AI ethics team at Google Research, and got her Ph.D. at Stanford from Feifei Li’s computer vision lab, so she’s no lightweight. I should disclose that I know Timnit, and we are members of the same professional association.
Long story short, there was an AI bias disagreement on Twitter centered on a celebrated white male giant of the field and a well-credentialed rising star of a black woman leading the fight to reform that same field's attitudes about the social consequences of its research. You can imagine how ugly things got. In the end, Yann LeCun quit Twitter, and Timnit Gebru's getting death threats from white supremacists.
Dear reader, if you are an ML researcher, you might be understandably exhausted about this debate and prefer to think about nice clean technical problems you can solve. I empathize.
I still think it is worth diving into this postmortem by The Gradient. If you read nothing else about the past few weeks, read this, I promise you won’t regret it.
Go deeper
Lessons from the PULSE Model and Discussion — The Gradient
AI Weekly: A deep learning pioneer’s teachable moment on AI bias — Venture Beat
This Image of a White Barack Obama Is AI's Racial Bias Problem In a Nutshell — Vice Motherboard
CVPR Tutorial by Timnit Gebru and Emily Denton on AI Ethics
One takeaway you might have missed:
AI-Startups will NOT curate their data.
LeCun’s assertion, echoed by many others, is that you can correct for bias against certain subpopulations in data by simply over-sampling groups that appear with low-frequency.

I’m a mathematical statistician by training. Something inside my soul screams at the idea that if there is bias in an inference, you can fix it by just mutilating the data to look like some platonic ideal. There is are some deep thoughts to be thought about this assertion, but let’s put it aside.
Let’s assume someone is going to this over-sampling. Does that mean that person is going to go in there and label all the black people? What about the other groups of people who might otherwise have a biased result? Who decides what groups are worth labeling and what the labels should be?
Think about the economics.
Consider the number of images that goes into training a modern deep neural network.
Consider how much man-hours it would take to do all of that annotation.
Ask yourself, who is going to pay for all of that?
Timnit Gebru argues that instead, the right way to things is to approach the collection and curation of data the way archivists approach collecting artifacts for historical archives.
That sounds even more expensive and time-consuming.
AI Startups will NEVER do this.
The economic and engineering realities of the people who are building and deploying computer vision apps is a critical point that gets lost in the controversy.
Speaking as a machine learning engineer in an AI startup, I promise you machine learning engineers in AI startups are NOT doing this.
Startups with limited runway don’t have the money or the time to spend on things like this
Engineers are under tremendous pressure to get something demo-able up and running fast-fast-FAST. Correcting bias in the data is the last thing they are thinking about.
Why focus on startups? Because they, not the big tech companies, are the ones who are selling computer vision to law enforcement.
Take, for example, Clearview.ai, which sells a giant searchable database of pictures scraped from social media to law enforcement. If your face has been online, it’s likely in their database. Their founder is Huon Ton-That. This image was on his Spotify page.
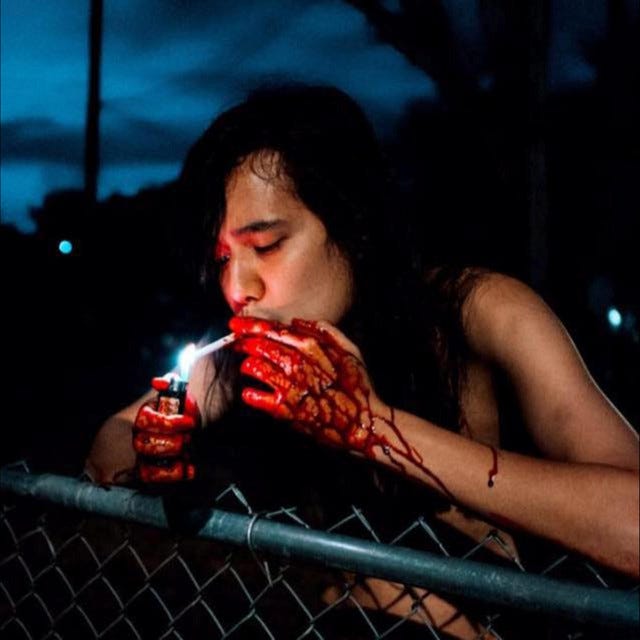
Prior to Clearview, he was known for a phishing scam. He and other members of Clearview have been tied to white supremacist and far-right causes. Further, Clearview must have violated countless online terms-of-service policies to compile that database.
I’m going go out on a limb and say these folks are not going to do Yann LeCun’s data manipulation or Timnet Gebru’s data curation.
Go Deeper
Facial Recognition Companies Commit to Police Market After Amazon, Microsoft Exit — WSJ
The Secretive Company that Might End Privacy as we Know It — NYT article on Clearview.ai
The Far-Right Helped Create The World’s Most Powerful Facial Recognition Technology — The Huffington Post
Clearview AI CEO Disavows White Nationalism after Exposé on Alt-right ties — The Verge
Another takeaway you might have missed:
Some categories are way too hard to categorize.
Here is another reason Yann LeCun’s suggestion of frequency tweaking won’t work.
Consider the case of this Marylin Monroe image.

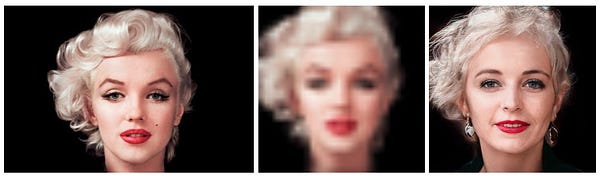
Note that when you, the human, look at the down-sampled image, you zoom in on all the things that make Monroe iconic. The subtle parting of the lips, the seductive gaze. Those little details are what make her so recognizable. The algorithm missed all those details. The algorithm constructs the upsampled image by looking at statistical patterns across the dataset. But it is not the population patterns that matter. It is those statistically negligible details that make Marylin, Marylin.
But then consider that it is small details we use as cues about things like race.
That’s why that image of Obama and AOC are so provocative. What elements of Obama’s and AOC’s faces make them POCs? Subtle ones. If an extraterrestrial were to land on Earth and was asked to start sorting humans according to our historically fluid definitions of race and ethnicity, how well would it do with people like Obama, AOC, and (ahem) me — people with mixed-race ancestry? Human culture puts a large emphasis on ignoring commonalities and picking out those details.
In a society that invented categories like “quadroon” and “octoroon,” one can have a lot of overlap with the Caucasian mode of the joint probability distribution of faces, while still having an identity and a set of experiences that is distinctly non-White.
What resampling technique is going to capture that extremely fraught bit of cultural nuance?