Ear to the Ground
Tech giants threw might behind foreign student expulsion debacle.
Last week, Harvard and MIT had sued the Trump administration over ICE rules that would rescind visas for international students who were taking exclusively online classes.
A few days ago, the administration caved and reversed policy minutes before the Boston federal judge was scheduled to hear the case.
Google, Microsoft, and Spotify filed a brief that threw their weight behind the Harvard and MIT lawsuit.

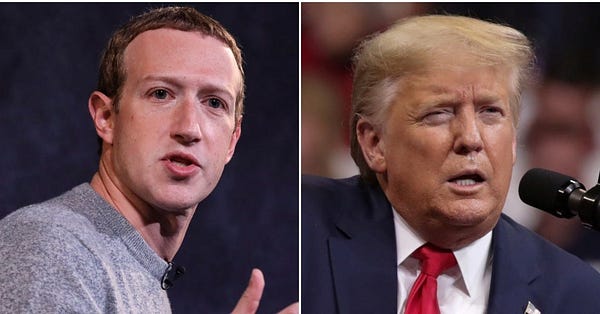
Foreign Students and Graduate STEM Enrollment — Inside Higher Ed
Google, Facebook, Microsoft, and other tech companies have joined MIT and Harvard in a fight to stop Trump's new visa rule — Business Insiders
AI for the Rest of Us
When does AI scalability matter?
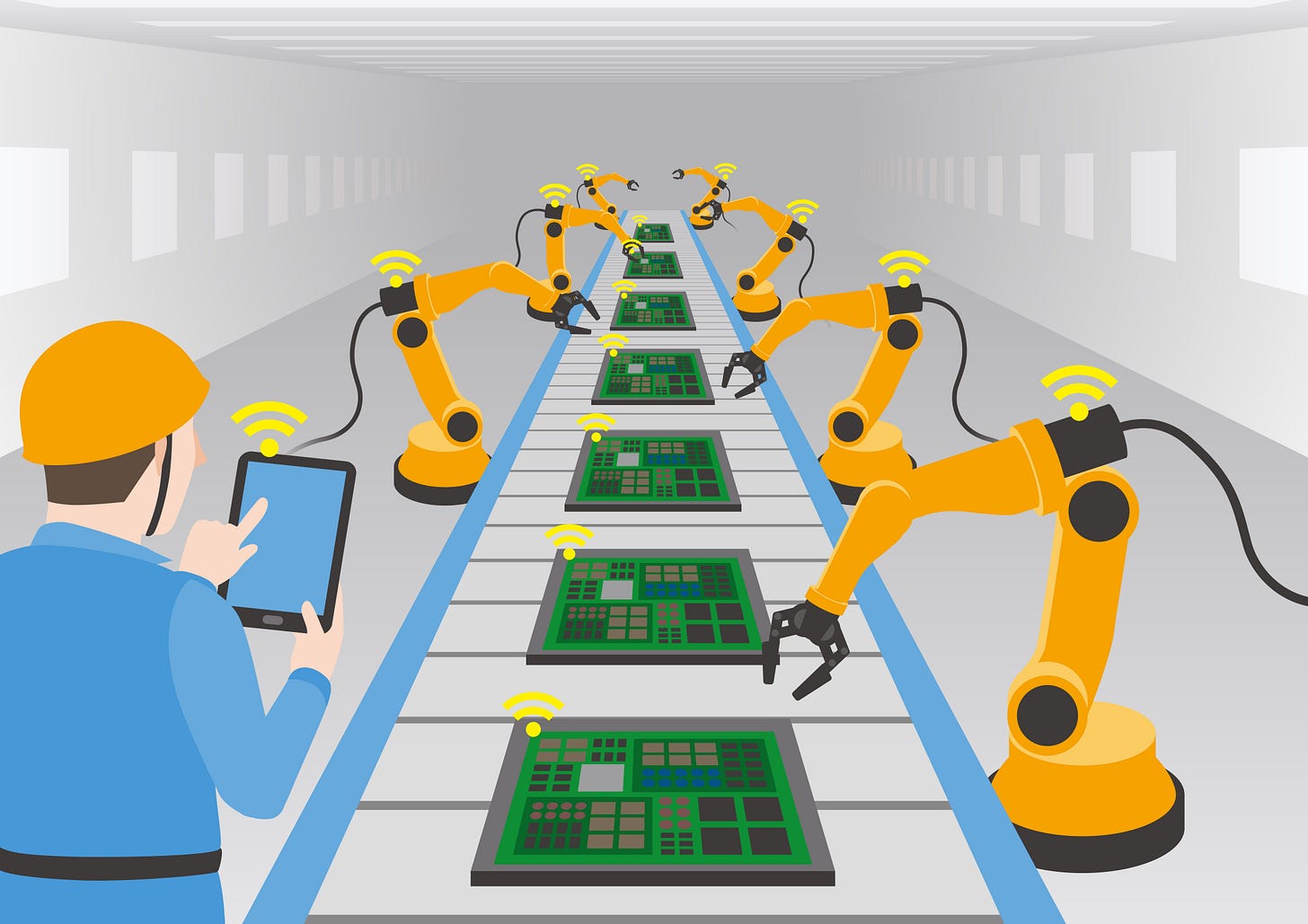
Execs who read AI news have learned to see beyond the surface hype of AI.
They likely have read investor views on the challenges of building AI products.
They may have also seen some articles and books that break down the technical challenges of AI.
A debate between AI experts shows a battle over the technology’s future - Technology Review
A Great Model is Not Enough: Deploying AI Without Technical Debt - DataKitchen
But tech journalists and AI thought leaders have little to say about the core unit economics of building an AI product. That is odd because the most important question one can ask when evaluating a product is whether or not the dollars add up.
Recently, I’ve been working with a friend and colleague, Glynnis Millhouse, to hash out some of these economics. Glynnis is digging into this topic as part of the research she’s conducting at Stanford GBS.
One key ingredient (more to follow) for building a financially viable AI product is identifying when scalability matters.
Scalability matters when it creates value (duh)
Suppose a university is contemplating building an AI-powered electronic discovery tool that would use advanced natural language understanding tech to speed up research.
More efficient research would be great for science, but how do numbers work for the university? Does it make sense to make the investment in building this tech when it has an abundant supply of effectively indentured grad students who will do the rote work of reviewing papers to gain their desired credential?
However, this same technology exists in drug discovery, with products like MetaCore. It exists for financial news in companies like Bloomberg.
Consider, for example, law firms. Companies like Everlaw are valuable because they increase the value that can be extracted from a billable hour.
The question is, when does scale matter?
There is excitement about scalability through automation, i.e., AI-applications where the following is true.
A skilled human can likely perform the task better than AI.
But the AI can do that job well enough, enabling automating away human labor, and hence scalability.
The scalability must matter.
Customer service is a good example of this, a la Solvvy. Another is the personal styling services of StitchFix. At StitchFix, the AI can’t pick styles as well as a skilled stylist, but they can do it well enough, and let StitchFix only charge $50.
Automated style-selection isn't merely an optimization for StitchFix; it allows the business to exist in the first place.
This seems obvious but…
We are seeing many cases of AI projects that check off 1 and 2 but not 3.
This analysis is part of some ongoing work by Glynnis and me. If you know anyone whose into back-of-the-napkin $$$ calculations on AI, please share.